Speech
recognition
glossary
Written by Dr. Amelia Kelly, our CTO
Speech recognition for education:
a glossary of key terms
As voice technology begins to emerge as an indispensable tool in the teaching toolkit, it is vital that school and district administrators become more comfortable with the technology and, in particular, its use with children in learning environments.
The voice technology that most of us are familiar with – i.e., the technology powering voice assistants like Alexa and Siri – was never designed with children in learning environments in mind, and even less so, children from underrepresented backgrounds.
This glossary of key terms was designed to help educate educators so that they can be more informed consumers of this exciting new technology, understanding its potential to support all learners, both remotely and in the classroom.
Glossary
Artificial intelligence (AI)
Systems designed to carry out tasks autonomously rather than being specifically programmed by humans.
Why it matters: AI is increasingly being used in education products, a trend that, no doubt, will continue in the coming years.
Machine learning
A subset of AI that trains computers on large amounts of data so they can carry out tasks automatically and at scale.
Why it matters: Machine learning algorithms “learn” and “improve” with each experience, which improves the speech recognition functions of voice-enabled educational tools.
Deep learning
A machine learning algorithm based on deep neural networks, which require large amounts of training data and have a multi-layered architecture that allows them to model complex behaviors like human speech and language usage.
Why it matters: Neural networks are used extensively for speech recognition, image recognition, and other pattern-recognition problems, which have applications for K-12 early literacy learning.
Voice technology
An umbrella term for technologies that allow users to interact with products, services, and platforms using their voices.
The underlying technologies that enable this are speech recognition (understanding human speech), speech synthesis (computers speaking aloud), natural language processing (reading and understanding human language), and machine translation (converting human speech from one language to another).
Why it matters: In the K-12 edtech context, voice technology—and speech recognition, in particular—can power a number of use cases, enabling independent reading practice, language learning, dyslexia screening, learning feedback, and summative and formative assessment.
Automatic speech recognition / speech recognition / speech-to-text
Allows digital devices to convert speech into text, making it easier for a device to understand the intent of the speaker. Words or concepts in the text can trigger actions (e.g., turn off the lights, text my sister).
Why it matters: Once a digital device has a transcript of the child’s reading, it can compare it against a rubric to determine reading fluency and comprehension. It can also provide time stamps for individual words, making it easy for a teacher to find a particular word or phrase read by the child, and listen back to it.
These language learning systems can also return pronunciation “confidence scores” at the utterance, word, and even down to the phoneme level.
De-biasing
Intentional processes used to reduce or remove unintended bias in speech recognition. Artificial intelligence systems can reflect the biases of their creators, resulting in inferior and often prejudicial experiences for under-represented users.
Machine Learning algorithms, in particular, carry out decisions based on data sets on which they have been trained and can become biased if those data sets are not representative of diverse populations.
Why it matters: A biased system can amplify and propagate deep-seated prejudices held by the designers of that system, as well as the limitations of available data sets. The effects of such biases in practice, assessment, and screening platforms, and in learning tools for kids can be disastrous.
If a biased system fails to understand a child’s accent or dialect while reading, for example, it can feed back to that child that they are a poor reader when, in fact, they’re reading correctly. An unbiased system, on the other hand, will offer fair and uncompromised feedback and data to facilitate education companies and platforms in supporting children on their learning journey.
Voice-enabled assessment
Uses speech recognition technology to listen, identify, and assess learning invisibly while the child is reading aloud.
Why it matters: Voice-enabled assessment tools used in the classroom and remotely, can provide data on pronunciation and oral reading fluency. They can also be used to screen for learning challenges like dyslexia.
When used to power assessments, speech recognition technology provides data that can support and improve educational outcomes for children, as well as help determine the type and level of support provided by teachers.
Keyword detection
A feature of speech recognition engines that identifies keywords and phrases in speech.
Why it matters: Keyword detection is particularly useful when analyzing children’s speech, where search terms in an audio file can be identified either in isolation, in a sentence or through background noise.
For example, a child might pick his or her favorite animal from a list. Keyword detection scores for each of the possible responses, triggering a response within the game or lesson.
Pronunciation assessment
Assesses the quality of the pronunciation of a word or phrase.
Why it matters: Pronunciation assessments are a tremendous time-saving tool for teachers, particularly when supporting in-person observational assessments because they provide teachers with scores that compare what the child actually said to a target given word, thus empowering teachers to better understand where a student may be struggling and need more support or attention.
Fluency assessment
Assesses children’s oral reading fluency.
Why it matters: Another time-saving tool for teachers. When a child reads a passage, the speech recognition system records and counts the number of word substitutions, omissions, insertions and correct words.
That, in turn, becomes a measurement of fluency measurement, sometimes expressed as “words correct per minute” or “WCPM.”
Speech-therapy assessments
Voice-enabled assessments that evaluate speech patterns and sentence structure.
Why it matters: Speech recognition-powered screening and practice tools can identify speaking patterns that may point to speech development pathologies enabling students to practice at home between speech therapy sessions, while also providing progress data to speech therapists.
Privacy-by-design
Privacy by design is an approach to technology development, design, and processes that ensures individual users’ data privacy rights are protected from the earliest stages through to the end-user experience.
Privacy by design commits companies to transparency when it comes to handling data, for example, a commitment to only use the data they collect to improve their service and not for any commercial purposes such as reselling, profiling, or advertising.
Why it matters: When it comes to kids’ data rights, privacy cannot be an afterthought or designed in at a later stage. Privacy needs to be baked into every level of infrastructure, data, and process, and be part of the ethos and vision of the voice-enabled solution from the very beginning.
Dr. Amelia Kelly
CTO
Dr Amelia Kelly is an artificial intelligence engineer and scientist specialising in automatic speech recognition of children’s voices. She is currently VP of Speech Technology at SoapBox Labs and holds a B.Sc. in Physics and Astronomy from NUI Galway, and an M. Phil and Ph. D in Linguistics and Speech Technology from Trinity College Dublin.
Amelia has more than a decade of experience in speech signal processing, natural language processing, machine learning and artificial intelligence. In her career to date, Amelia has held various positions in industry and academia, including with IBM Watson, in Silicon Valley, and as a research fellow at Trinity College, Dublin. As well as various academic publications, she holds a patent in the area of cognitive computing and is a regular speaker at international technical conferences and industry events. She is a Fulbright TechImpact Scholar for 2020/2021.
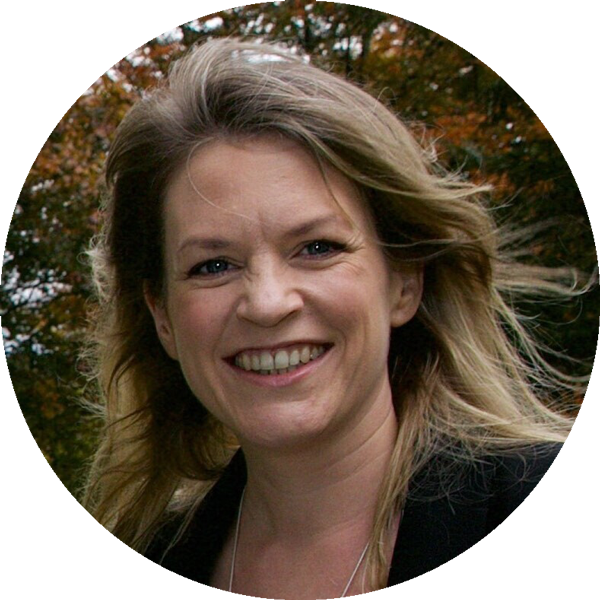